Faster, Scalable IoT Anomaly Detection of Real-time Data Streams
- Robert Lutz
- Mar 18, 2022
- 2 min read
Updated: May 26, 2023
Today’s businesses are increasingly reliant on digital technologies for almost every aspect of their business. Many are using, or are exploring, machine learning to unlock deeper insights that enable smarter decision-making toward their goals. Making good decisions from machine learning enables businesses to be more objective and proactive in decisions that can be defended with data insight evidence.
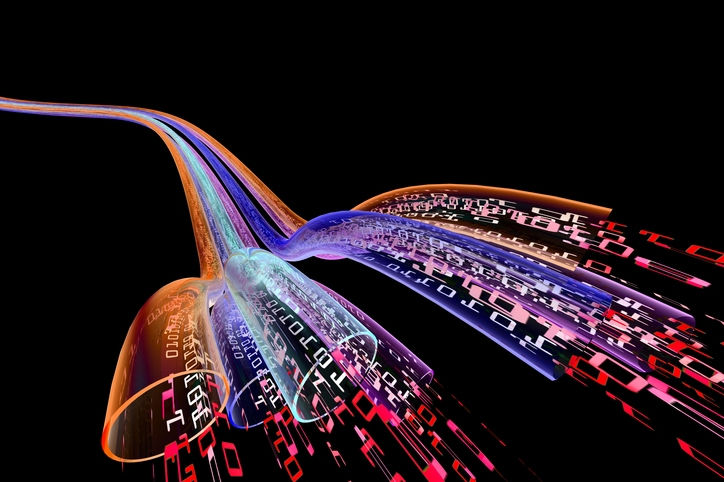
The amount of data available, its characteristics, and the speed at which it is collected is growing exponentially. There is a growing need to extract deeper insights from data at faster rates that can scale quickly while keeping costs low. Real-time analysis is helpful for monitoring devices for failures, loss of critical functions, errors, cybersecurity issues, and more. This will lead to faster decisions and solutions that result in happier customers and lower costs.
Traditional Machine Learning
Machine learning typically uses a large batch of data collected and stored over time. The machine learning process is executed periodically, perhaps hourly or daily. Often, some level of human intervention is required to handle and interpret the results. This traditional machine learning solution does not lend itself toward real-time analysis, and there are costs associated with the storage and movement of large amounts of data.
What is OTA-ML?
Unlike batch processing, OTA-ML applies transactional machine learning to real-time data streams. Data streams are continuous flows of data that change quickly over time.
OTA-ML analyzes streaming data with the ability to look back or rollback the data stream as a window in time. This rollback can be 10 seconds, 30 seconds, or any length of time required. The rollback happens continuously from the latest data point to whatever time is necessary. This sliding window offers important information and can be used to dynamically (and automatically) build training datasets for machine learning. This is a critical advantage of OTA-ML -- the speed in analyzing data, building training datasets for each individual device, applying transactional machine learning, and then using the trained algorithm for predictive or prescriptive analytics. OTA-ML operates much faster and more efficiently than traditional machine learning so it can keep up with the demands of streaming data.
OTA-ML applies advanced algorithms in the sliding data windows such as gradient boosting, linear regression, logistic regression, and ridge regression. All parameters in these algorithms are automatically hyper-tuned. This means machine learning training datasets and tuned algorithms can be built on the fly for every individual device. For example, if there are one million IoT devices, OTA-ML can, if necessary, build one million machine learning models. This is not possible with conventional machine learning. OTA-ML can also build classification models that predict the probability of an event occurring.
Real-Time predictions are possible with OTA-ML. The ML models can be used instantly after being deployed for predictive analytics.
Example of OTA-ML in Action
As a simple demonstration, we applied OTA-ML to analyze real-time IoT devices for anomalies, as shown in the figure below. This is a live dashboard reporting the analyses of thousands of IoT devices for voltage, power, and current issues. It highlights devices exhibiting anomalies on the map and generates real-time alerts.
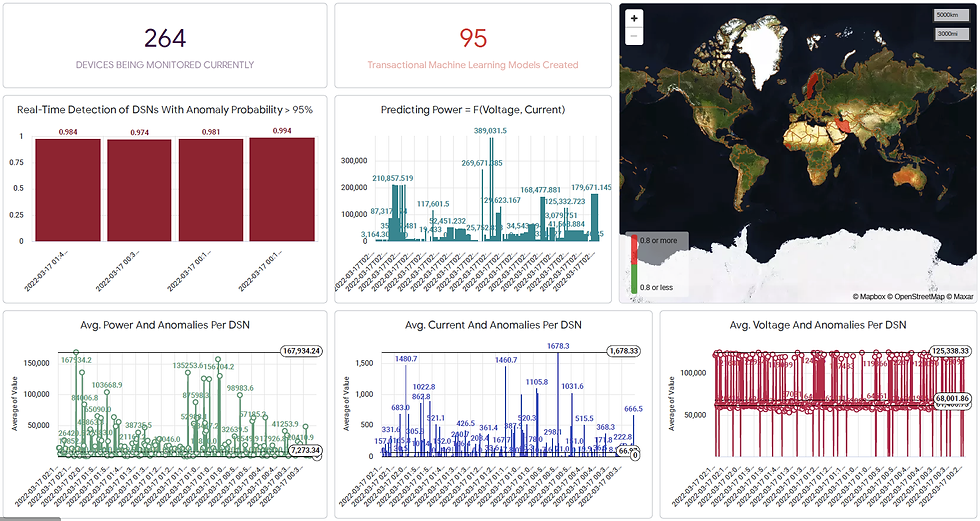
Businesses looking for faster and cost-effective insights into their IoT data should consider using OTA-ML and transactional machine learning at scale. Let the experts at Ayla Networks show you how.
Comments